For a while now, I’ve been working on making Blurity faster. It’s come a long way since the first public release. What once took 6 minutes now takes about 10 seconds. That increase in speed has come from a variety of factors, including rewriting all of the image processing code in multithreaded C++ and having several ground-up algorithm redesigns. Unfortunately, the newest version of the blur modeling code suffered from poor concurrency. I could throw about three worker threads at it, but beyond that there were sharply diminishing returns. As in no returns. I’d throw more threads at the problem, and I wouldn’t see any improvement in the completion time.
I had implemented a basic producer-consumer pattern in the blur modeling code. I wanted to be able to support server instances with a variable number of cores, and since I knew it was bad to have more running threads than cores, it seemed like a reasonable choice to have a variable number of consumer threads servicing the work queue. That work queue had a constant number of items in it when filled: five virtually identical items and one item that would require slightly less processing. The boss would be called and fill the queue with six items, then the workers would consume the items, and the boss would wait for the queue to be empty and all of the workers to be done before returning.
When I first noticed that having more than three worker threads seemed to bring no increase in performance, I came up with some crazy hypotheses about the cause. For a while I was even thinking that calls to malloc() and its equivalents were somehow causing the worker threads to block one another, but that, of course, was not happening. Then I got out Valgrind, a powerful profiling tool, and gathered data about where the app was spending its time.
In spite of overwhelming evidence that the program really was dominated by arithmetic related to FFTs, I somehow convinced myself that I was dealing with a cache problem. Several days were waisted trying to eliminate the handful of spots in the parallel code that were experiencing ~1% L2 cache misses (that was as bad as it got, fortunately). At one point, I was even thinking that the scheduler was doing a bad job of keeping threads on the same core, thus supposedly causing the cache to be unnecessarily invalidated, so I started looking into sched_setaffinity() and other such nonsense.
That mucking around didn’t help matters from a cache-hit perspective (and in many cases made things worse — thank you, version control!), but it did lead me to discover I had been using FFTW in an inefficient way, so the time wasn’t totally wasted. In fact, fixing my FFTW gaffe led to a 40% improvement in the blur modeling algorithm execution time — but there was still no improvement when using more than three worker threads.
The actual cause of the problem struck me today when I was, of all things, calculating the incremental price per bagel for a potential multi-bagel purchase at Panera. I realized that it was simply a matter of factorization, and I immediately felt stupid.
Remember that there are always six work items that start in the queue. If the number of consumer threads is a factor of the number of work items, and the work items all take roughly the same amount of time to process, then the queue will be emptied with little to no wasted time.
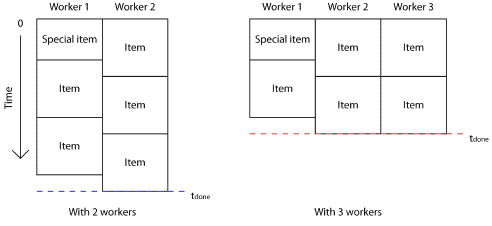
Going from 2 to 3 worker threads decreases the total time (t_done)
However, if the number of consumer threads is not a factor of the number of work items, the queue will not be emptied efficiently, and time will be wasted waiting for one or more of the threads to finish after the others are done.
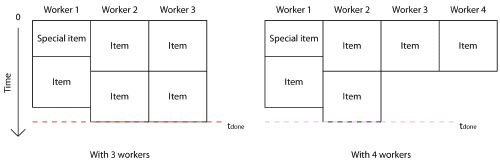
Going from 3 to 4 worker threads does not change the completion time
All of this is blindingly obvious in hindsight. Since 1, 2, and 3 are all factors of 6, it comes as no surprise that adding threads to have 2 or 3 total workers leads to speed improvements. Likewise, since 4 and 5 are not factors of 6, it should have been no surprise that having those numbers of worker threads led to no speed improvements.
That meant that the next major speed boost should happen at the next higher factor of 6: namely, 6 worker threads. Indeed, that’s what we see in the execution times.
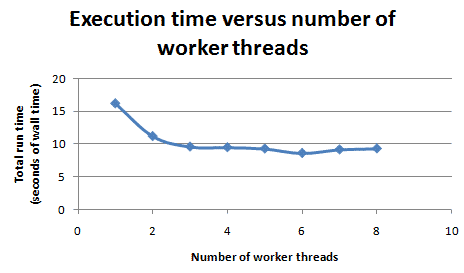
Execution times as function of number of worker threads (lower is better)
Three things to note on that chart:
First, there are diminishing returns. Going from 1 to 2 worker threads leads to a 31% improvement, but going from 2 to 3 worker threads gives only an additional 14% improvement. Going from 3 to 6 worker threads improves times only 10% more. In addition, this suggests that other parts of the code — outside of the threaded producer-consumer block — are potential targets for optimization. That is because the gains from each thread are not as high as we’d expect if the work items dominated the overall execution time.
Second, there is a very slight improvement in execution time with 5 threads, about 3%. This is because of the previously noted “special” work item, which takes slightly less time to process than all of the other work items. In the 5-thread case, the thread that gets two work items will always have the special item and a normal item, whereas in the 4-thread case one of the threads would have two normal items, thus the time difference.
Third, the execution time actually gets worse as the number of threads increases beyond 6. There are never more than 6 work items in the queue, so those extra worker threads are just added overhead.
(All of these measurements were on the same idle 8-core machine.)
In conclusion, I should have recognized this now-obvious situation earlier. I guess I simply overlooked the slight dip with six threads. Now that I do see the high-level cause, I can tell that throwing more hardware at the problem is not a good use of money; a 10% speed improvement for double the cores is a bad return on investment. The other parts of the code, or the algorithm itself, will need to be improved in order to see significant gains.
Oh, and the rule still applies.
Recent Comments